iPIM - Machine Learning for Predictive Maintenance of Pipelines
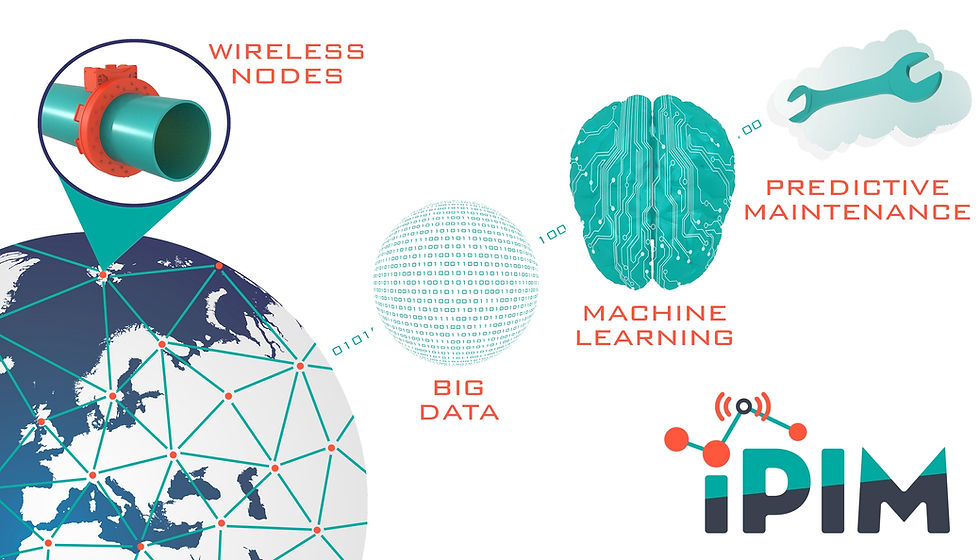
The Intelligent Pipeline Integrity Monitoring System (iPIM) Is a Structural Health Monitoring (SHM) system for pipelines. The system applies machine learning to predict pipeline failure before it occurs in time for preventative maintenance.
What is Machine Learning?
Machine learning is a form of artificial intelligence (AI) where the computer learns through statistical analysis of input data to predict the outcome. As the computer is fed with more data input the accuracy of the prediction increases. This process takes place automatically without need for explicit programming.
Here is a short video which gives an excellent demonstration of a machine learning application where the computer learns how to navigate Mario through a stage of Super Mario World;
How is Machine Learning Applied to Pipeline SHM?
The iPIM system data inputs are received via a network of permanently installed wireless nodes. Each node uses a dual mode ultrasonic technique to constantly monitor for cracks, corrosion and other faults over large sections of pipeline. The data is sent over a wireless communication system to be processed by the AI machine learning algorithm.
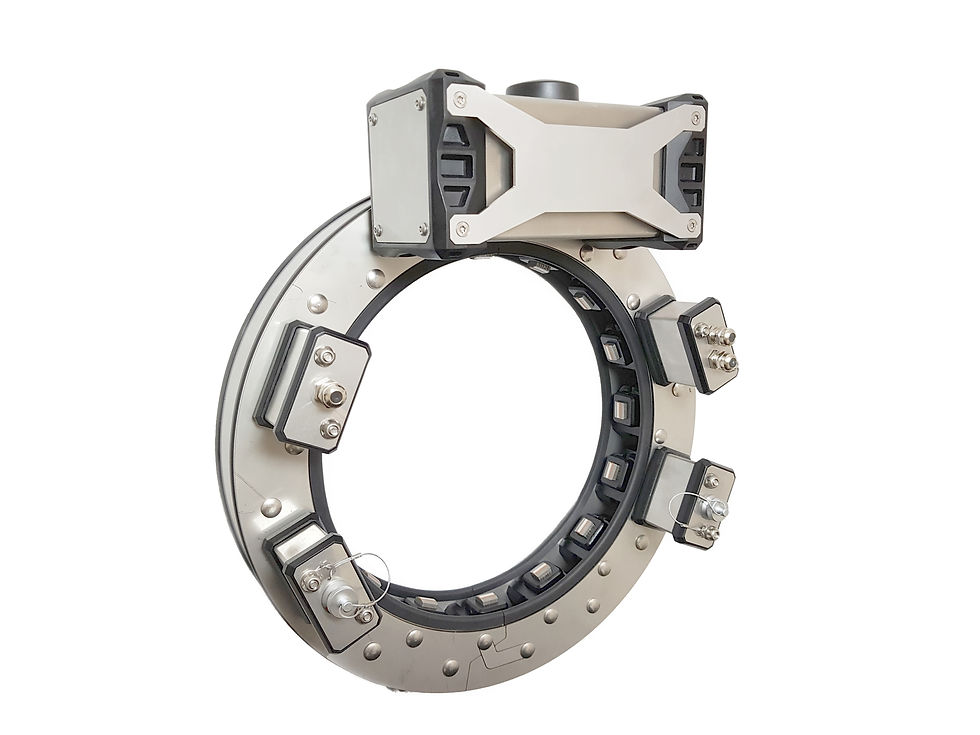
What are the Advantages of Machine learning for SHM?
The resolution of the fault-finding capability increases through long term gathering of “big data “and machine learning on particular conditions of a specific pipeline. This is the advantage over conventional inspection methods that can only have a snapshot of the condition and only guess the growth of the fault in-between inspections.
How does the iPIM System Predict Failure?
By applying Machine Learning and continuous monitoring of specific areas of the pipeline you can have access to accurate information about the specific conditions of a particular pipeline in certain environmental conditions. This is achieved by monitoring the pipeline over time and fine-tuning the interpretation of the data for accuracy. By monitoring the condition of the corrosion or other faults and their growth rate over time the system will achieve precision predictive maintenance.